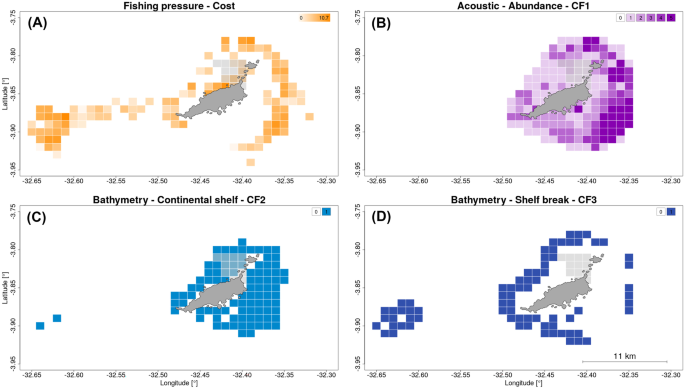
Reserves are at the heart of global policies to stop the erosion of biodiversity. Optimisation is increasingly used to identify reserve locations that preserve biodiversity at a minimum cost to human activities. Two classes of algorithms solve this reserve site selection problem: metaheuristic algorithms (such as simulated annealing, commonly implemented in Marxan) and exact optimisation (i.e. integer programming, commonly implemented in PrioritizR). Although exact approaches are now able to solve large-scale problems, metaheuristics are still widely used. One reason is that metaheuristic-based software provides a set of suboptimal reserve solutions instead of a single one. These alternative solutions are usually welcomed by stakeholders as they provide a better basis for negotiations among potentially conflictive objectives. Metaheuristic algorithms use random procedures to explore the space of suboptimal reserve solutions. Therefore, they may produce a large amount of similar, thus uninformative, alternative solutions, which usually calls for a heavy statistical post-processing. Effective methods for generating a diverse set of near-optimal solutions using exact optimisation are lacking. Here we present two new approaches for addressing this issue. Our algorithms explicitly control both the optimality gap and the dissimilarity between alternative reserve solutions. It allows the identification of a parsimonious, yet meaningful set of reserve solutions. The algorithms presented here could potentially increase the uptake of exact optimisation by practitioners. These methods should contribute to less noisy and more efficient discussions in the design of conservation policies.
DOI: doi.org/10.1007/s10666-022-09862-1
Reference
Brunel, A., Omer, J., & Lanco Bertrand, S. (2022). Producing a diverse set of near-optimal reserve solutions with exact optimisation. Environmental Modeling & Assessment, 1-16.